Loading...
First I just require some code I have previously written. (I guess I really should make them into gems or something instead of copying code around...) names.rb tries to guess sex from a person's name and/or title, and countries.rb maps countries to continents, regions etc with some fuzzy matching of names. (I'm looking at you Democratic Republic of Kongo, Koreas etc.) Easy-peasy.Then I set some standard variables. (Maybe I'll make this dynamic in the future, why not?) The most interesting entry here is the classifiers hash. This maps the columns we want to use later on to maximize the difference of to their respective weights. (This should of course be updated to match whatever is in the input file...) Another semi-useful parameter is number_of_runs. This is the number of simulations the partitioner will perform before keeping the best partitioning. And of course number_of_groups - the number of groups that the students will be split over.
count is just a variant of my count function already covered here in the blog (link).
I slurp up the list of participants as an array of hashes, from the file name specified above, converting the headers as specified in the variable_names_map hash and generating some standard variables in the process - unless they are already present.
The important function in my naive approach is the scoring_function. This basically looks at the extremes one variable at the time. That is, it checks to see if any value (or category) of a variable is over or underrepresented, based on the expected number of elements with this value. samples is a count of number of elements in the group. I iterate over all categories of the variable to find the worst sinner (squared). So if we are looking at sex, it will check male, and then female and generate a score based on the category that is most off from the expected value (The number of participants with that characteristics divided by number of participants in this group).
The penalty_function simply assigns scores to each group in the current partition using the scoring_function per classifier multiplied by the weight of the respective classifiers.
The main loop of the program runs the simulations number_of_runs times.
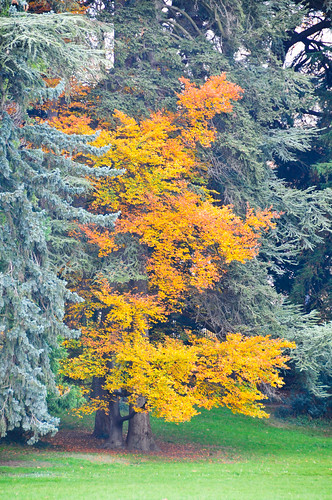
After this loop I just write out the best partition.
Voila - it takes about
The group work got done and groups seemed fairly balanced.
Limitations:
- Penalizes small groups more heavily than larger groups (if some groups has more participants than other)?
- Variables with more categories dominates the score? (There's probably a useful statistical tool to solve this... (Normalisation?))
- Variables have to be categoric (age has to be grouped).
Potential future work:
- Split out the scoring function (and possible the penalty function) as a stand alone library.
- Let the script take arguments for useful parameters (filename, number_of_runs, group_size, variable_names_map, classifiers etc...)
- Could it be interesting to look at continuos variables (ie. age) as well as categorical ones?
- Rather than discarding previous partitions one could let new ones evolve from a certain number of branches? (Local minimum problems?)
(BTW; God jul!)